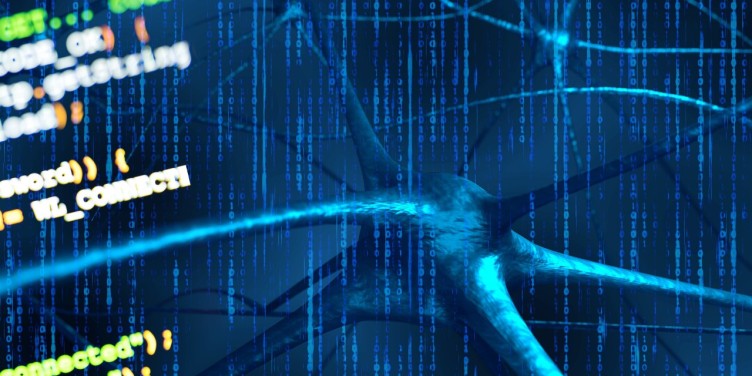
Artificial Intelligence in Drug Development – Part 1: The Basics…A Primer on the Many Different Types of AI
Stephanie Pasas Farmer, PhD
President and Founder at Ariadne Software
Blog Series:
Artificial Intelligence, or AI, is the watchword for innovation in drug development, something that is needed now more than ever. While AI is rapidly evolving, there’s plenty of education needed and myths to dispel. This 3-part series defines AI, explores how it is used in drug development and looks to the future as standards and technologies mature.
Part 1: The Basics on AI … A primer on the many different types
Background
If you work in drug development, you’re probably inundated with provocative news headlines involving artificial intelligence (AI), lauding its potential for transformative impact from discovery through clinical development and post-approval studies. For every benefit claim, there are ample amounts of warning calls to ensure the industry doesn’t make it a solution in search of a problem. Although these are early days, progress is real, and there are scores of AI applications in use across every stage of drug development.
The two major benefits of AI include (1) a drastic reduction of time-intensive and costly tasks through automation, as well as (2) improvement of the scientific quality of data evaluations, leading to improved decision-making and success rates. The potential for data quality improvement has significant implications in early drug development, clinical outcomes, safety signals and real-world evidence. When you consider the high rates of failure in drug development, combined with unprecedented amounts of data and average cycle times required for clinical development, AI technologies offer transformative promise.
Key Technologies and How They Work
There’s a lot of technology, terminology and categories to tussle over, in gaining understanding how they can be applied to drug development and advance efficiency, speed and precision. If you’re confused by the ever-expanding and often conflicting AI definitions and categories, you’re not alone. The fluidity of definitions and new categories make confusion easy for even the most knowledgeable of tech insiders.
As background context, there are several defining categories within the AI framework. The first is weak AI, which is designed to focus on a specific or narrow task. It gives the appearance of intelligence but operates in a rules-based context, meaning the applications only know what to do in the situations that they are programmed for. Strong AI, on the other hand, emulates a real human mind, without predefined algorithms, and with the power to learn from experience and change its behavior. However, strong AI, whose potential is vividly depicted in the movies and TV shows, does not yet exist and won’t for some time to come.[1] Even though we do not have access to strong AI yet, notable and eagerly watched advances have been made in the field of machine learning, which involves decision-making from inference and learning.[2]
Common applications include:
Expert systems may be the simplest form of AI. It emulates decision-making in a field that typically requires a subject matter expert, based on programmed facts and rules. An inference engine provides a methodology for reasoning about information provided. In drug development, expert systems help with classification, diagnosis, monitoring and/or data comparison as well as prediction.[3]
Machine learning enables computer systems to learn by doing, building on that experience without being explicitly programmed. It is common for machine learning applications to use past data to predict behavior for future events. Machine learning incorporates levels of supervision, with varying amounts of reference data to help determine patterns, relationships and structures, typically on more granular levels than humans are capable of. Machine learning works well with large amounts of data.[3] Common examples include using genetic data to discover novel biomarkers; or using preclinical and clinical data to predict efficacy and side effects of specific drugs currently in development with existing rich sources of data.[4]
The artificial neural network is a common machine learning model. Artificial neural networks aim to emulate the way the human brain organizes information in order to make decisions and predictions. Within an artificial neural network, there are input, output and hidden layers. Information received is processed through multiple hidden layers, made up of artificial neurons. The hidden layer in between aims to transform the data into useful application by the output layer. As learning takes place and patterns recognized, the relationships and connections between these neurons are improved for predictive intelligence. Artificial neural networks are used for complex tasks as speech, object and image recognition.[5]
Deep learning is a specialized form of machine learning. It’s the process of understanding large volumes of data within multiple layers in a neural network, increasing computing power over large and complex datasets. Deep learning applications are in their infancy within drug development but at the very least are making news in drug discovery and biomarker development.[6]
Natural language processing (NLP) is one of the most widely used applications. In the consumer product world, think Siri & Alexa. In drug development, basic NLP techniques are used to help answer specific problems and range from text-mining of structured or semi-structured data (think scientific literature scans, adverse event data or electronic health record databases). The database of research information is run through an NLP application with focused topics, specific phrases, or words for it to search for. Text-mining not only identifies facts, it analyzes to determine contextual information. More advanced NLP techniques include the use of algorithms that can be applied to search for patterns of significance. which can considerably streamline data analysis in virtually every clinical trial function. [7] [8]
When paired with machine learning and/or expert systems, NLP can adapt to the specialized language it encounters. This allows a system to pick up a new set of vocabulary or conventions used in highly technical fields, giving scientists access to this technology to assist with their research.
In Part 2, I will outline some of the current uses in drug development, from discovery to late stage clinical development. Part 3 discusses the future of AI in drug development.
References:
[1] https://www.techopedia.com/definition/31621/weak-artificial-intelligence-weak-ai
[2] https://certes.co.uk/types-of-artificial-intelligence-a-detailed-guide/
[3] https://azati.ai/the-return-of-expert-systems/
[4] https://deepsense.ai/machine-learning-in-drug-discovery/
[5] https://www.datascience.com/blog/introduction-to-machine-learning-algorithms
[7] (https://emerj.com/ai-sector-overviews/natural-language-processing-in-pharma-current-applications/).