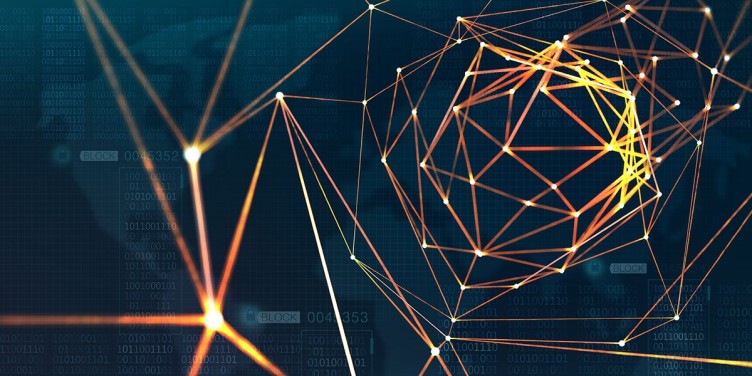
Artificial Intelligence in Drug Development – Part 2: It is real and happening now
Stephanie Pasas Farmer, PhD
President and Founder at Ariadne Software
Blog Series:
Artificial Intelligence, or AI, is the watchword for innovation in drug development, something that is needed now more than ever. While AI is rapidly evolving, there’s plenty of education needed and myths to dispel. This 3-part series defines AI, explores how it is used in drug development and looks to the future as standards and technologies mature. In Part 1, we set the stage by talking about categories, types and technologies in AI.
Part 2: It is real and happening now
AI in Drug Development
AI in drug development is focused on efficiency, automation and predictive analytics. The most concise summary of the role of AI in drug development came from a not-so-recent article in Clinical Informatics quoting Josh Stanley, Product Manager who said “It’s not to replace, but to augment and assist human intelligence, incorporate user-friendly efficiency, leverage data and make predictions in clinical trials to detect trends and outcomes.” So, how is the biopharmaceutical research community using these technologies? Following is a summary of AI applications used in drug development. While it’s intended to be representative, it’s by no means exhaustive. New innovations are sprouting up every day.
Drug Discovery/Early Development: The identification of important links between genes, biomarkers, diseases and drug sensitivity, for the selection of the most promising compounds and even drug repurposing. AI can efficiently combine, scan and link information from literature, genomics and genetics, biological assay databases to connect drug, target and disease data and deepen our collective understanding of the biology of targets and diseases to improve target selection, target prioritization, biomarker discovery.[1] There are more than one hundred start-ups focused on AI applications in drug development with the lions’ share focused on discovery and early development, including innovators such as Insilico Medicine, Benevolent AI, Vyasa and Exscientia.
Bioanalysis: Bioanalysis is a particularly challenging area not just because of its high-volume data analysis, but also due to the amount of disparate data that needs to be linked and contextualized in critical functions of assay development and sample analysis. Data review for regulatory compliance remains a manual process for the research community and regulatory agencies, with a limited selection of tools for assistance. Even the gold standard of laboratory information management is still semi-automated, requiring manual re-entry of summary data from raw data. Ariadne Solutions’ Red Thread tool addresses these challenges by automating data review and connecting data across multiple supporting data tables to ensure safety, efficacy and scientific consistency. Data summary and contextualization from multiple tables is a common source of quality failures.
Optimization of clinical trial operations: Clinical trial feasibility, clinical site selection and patient recruitment are huge bottlenecks for successful clinical trials. AI techniques can identify and characterize patient populations and sub-populations best suited for specific drug classes.[2] Advances in analytics have transformed traditional approaches for trial feasibility and site selection, by analysis of historical site performance against eligibility criteria and access to the target patient population. This is a far more predictive tool than the traditional approach of investigator questionnaires.
Patient Recruitment: In patient recruitment, AI can identify suitable patients across different data sources (such as comparing electronic health records and other patient information such as imaging data with eligibility criteria) and support patient adherence to protocols once they are enrolled. After enrollment, AI can be a more reliable predictor of dropouts by analyzing patient-level data sources. For example, Antidote, a specialty provider of patient recruitment for global clinical trials, offers a clinical trial matching platform built on data from public sources such as clinicaltrials.gov. It standardized clinical trial terminology used on those sites into a structured language built for a search engine, providing more a precise search using trial eligibility and other criteria, such as distance potential participants are willing to travel.[3]
Adherence: Once a trial begins, adherence is the next big hurdle. Although researchers already have several options for estimating adherence, such as pill counting and medication containers that record and track administration, these methods cannot confirm actual intake. One novel application from AiCure involves computer vision technology to visually confirm medication ingestion. Using a mobile device camera, software algorithms confirm the identities of the patient and the medication and verify intake.
The app sends this information to a cloud-based dashboard that researchers or health care providers can use to monitor adherence and identify issues in real time. Providers can communicate with patients through the dashboard to offer immediate assistance. The application also gives interactive instructions, reminders and suggestions to patients to further increase adherence.
Patient-specific models capture deviations in “normal” behavior across facial or audio expressivity, movement, concentration and cognition.[4] What’s promising for current drug development efforts is the applicability of these models across all disease areas.
Safety Surveillance: Safety surveillance is an age-old challenge in drug development. Adverse event cases are often reported manually from a range of sources including post-marketing studies, patients and healthcare professionals around the globe, in multiple languages. The process is time-consuming and expensive, requiring medical monitoring expertise for analysis, coding and reporting within short durations to regulatory authorities. Machine learning applications can automate adverse event processing through a combination of text-mining, speech review, data extraction and codification. When manual review is needed, expedites data review by flagging potential low-quality data entries.[5] AI techniques hold even greater promise for signal detection through high sensitivity and predictive analytics.
In Part 3, we will discuss the future of AI in drug development.
References:
[2]https://www.sciencedaily.com/releases/2019/07/190717142716.htm
[3]https://www.wired.com/story/meet-the-company-trying-to-democratize-clinical-trials-with-ai/
[5]https://www.genpact.com/insight/case-study/transforming-drug-safety-through-ai